By Balakrishna D.R., Executive Vice President, Global Services Head, AI and Industry Verticals, Infosys
In recent years, artificial intelligence (AI) has rapidly transformed industries and societies, promising unprecedented efficiencies and innovations. However, as AI systems become more pervasive and powerful, there’s a growing recognition of the need for responsible development and deployment. While many organisations have embraced ethical AI principles, a significant gap remains between these aspirational guidelines and their practical implementation. Bridging this divide is crucial for realising AI’s full potential while mitigating risks and fostering public trust.
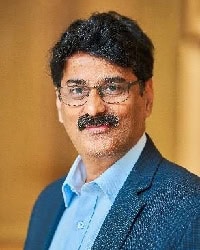
The Principle-practice gap
Numerous organisations and governments have published AI ethics frameworks, often emphasising values like transparency, fairness, accountability, and privacy. Yet translating these high-level principles into tangible practices has proven challenging. This challenge stems from various factors, including the complexity of AI systems, the rapid pace of technological advancement, and the multifaceted nature of ethical considerations across different contexts and applications.
For instance, while ‘fairness’ is a commonly cited principle, its practical implementation raises complex questions: What constitutes fairness in each scenario?
How do we measure and ensure it across diverse user groups? Similar challenges arise with other principles like transparency and accountability, where the technical intricacies of AI systems can make straightforward explanations or attributions difficult.
Operationalising responsible AI
To close this gap, organisations need to adopt a holistic approach that integrates responsible AI practices throughout the AI lifecycle. Key strategies include:
Contextualised implementation: Responsible AI isn’t one-size-fits-all. Organisations must tailor their approach based on the specific AI application, industry context, and associated risks. For instance, an AI system in healthcare might prioritise explainability and privacy, while one in financial services might focus on fairness and bias mitigation.
Cross-functional collaboration: Implementing responsible AI requires input from diverse stakeholders, including data scientists, ethicists, legal experts, and domain specialists. Collaboration across departments ensures a comprehensive approach addressing technical, ethical, and business considerations.
Continuous monitoring and improvement: Responsible AI is an ongoing process, not a one-time checklist. Organisations should implement robust monitoring systems to track AI performance, identify potential biases or errors, and continually refine their models and processes.
Ethical AI governance: Establishing a centralised responsible AI office or team can help coordinate efforts, develop and enforce policies, and ensure consistent application of ethical principles. This governance structure should have clear mandates and authority to influence AI development and deployment decisions.
Tools and frameworks: Utilising specialised tools and frameworks can facilitate the implementation of responsible AI practices, such as toolkits for detecting and mitigating bias in machine learning models or frameworks for transparent model reporting.
Implementing responsible AI
While all of this sounds great, the question remains: how can you implement these practices in your organisation? Several strategies have proven effective for adopting responsible AI practices.
One approach is to establish AI ethics committees. These cross-disciplinary teams provide guidance on AI ethics and influence product development, ensuring ethical considerations are included at every stage of AI development.
Another strategy is using AI fairness tools. These technologies assess and reduce bias in AI systems by analysing datasets and model outputs, helping ensure that AI systems operate fairly.
Ethical design frameworks offer guidelines for embedding ethics into intelligent systems, covering data rights and algorithmic bias to ensure ethical principles are central to AI design.
Lastly, responsible AI frameworks integrate ethical considerations throughout the AI lifecycle, including impact assessments and continuous monitoring. These frameworks help ensure that AI systems align with ethical standards.
Challenges and future directions
Despite advancements, challenges remain in implementing responsible AI. A key issue is balancing innovation and ethics. Organisations must navigate the tension between rapid AI development and ethical considerations, ensuring that progress does not compromise integrity.
Regulatory compliance is another challenge. As AI regulations evolve, organisations need to stay agile and adapt their practices, requiring vigilance to meet new laws and standards.
Measuring impact adds complexity. Creating quantifiable metrics for ethical AI performance, especially for intangible principles like societal benefit, is difficult. Clear indicators of ethical performance are vital for assessing the effects of AI.
Lastly, there is a growing demand for talent in AI ethics. Professionals skilled in both AI technology and ethics are sought after, necessitating new educational programs to prepare the next generation of AI practitioners to integrate ethical considerations into their work.
We must transition from merely stating ethical principles to embedding them within organisational cultures and practices. This requires technical solutions and a shift in AI development. Bridging the gap between principles and practice allows us to harness AI’s potential while mitigating risks, fostering public trust, and ensuring AI serves societal interests. Collaboration among technologists, ethicists, policymakers, and the public is crucial for a responsible AI-driven future.